What's Wrong with Social Science and How to Fix It: Reflections After Reading 2578 Papers
- 1. They Know What They're Doing
- 2. ...or Maybe They Don't?
- 3. There Are No Journals With Strict Quality Standards
- 4. Things Are Not Getting Better
- 5. Everyone is Complicit
- 6. Just Because a Paper Replicates Doesn't Mean it's Good
- 7. Lack of Theory
- 8. There's Probably a Ton of Uncaught Frauds
- 9. Power: Not That Bad
- 10. Field of Dreams
- 11. Limited Political Hackery
- 12. Interaction Effects Bad
- 13. Nothing New Under the Sun
- 14. What To Do
- 15. ...My Only Friend, The End
I've seen things you people wouldn't believe.
Over the past year, I have skimmed through 2578 social science papers, spending about 2.5 minutes on each one. This was due to my participation in Replication Markets, a part of DARPA's SCORE program, whose goal is to evaluate the reliability of social science research. 3000 studies were split up into 10 rounds of ~300 studies each. Starting in August 2019, each round consisted of one week of surveys followed by two weeks of market trading. I finished in first place in 3 out 10 survey rounds and 6 out of 10 market rounds. In total, about $200,000 in prize money will be awarded.
The studies were sourced from all social science disciplines (economics, psychology, sociology, management, etc.) and were published between 2009 and 2018 (in other words, most of the sample came from the post-replication crisis era).
The average replication probability in the market was 54%; while the replication results are not out yet (250 of the 3000 papers will be replicated), previous experiments have shown that prediction markets work well.1
This is what the distribution of my own predictions looks like:2
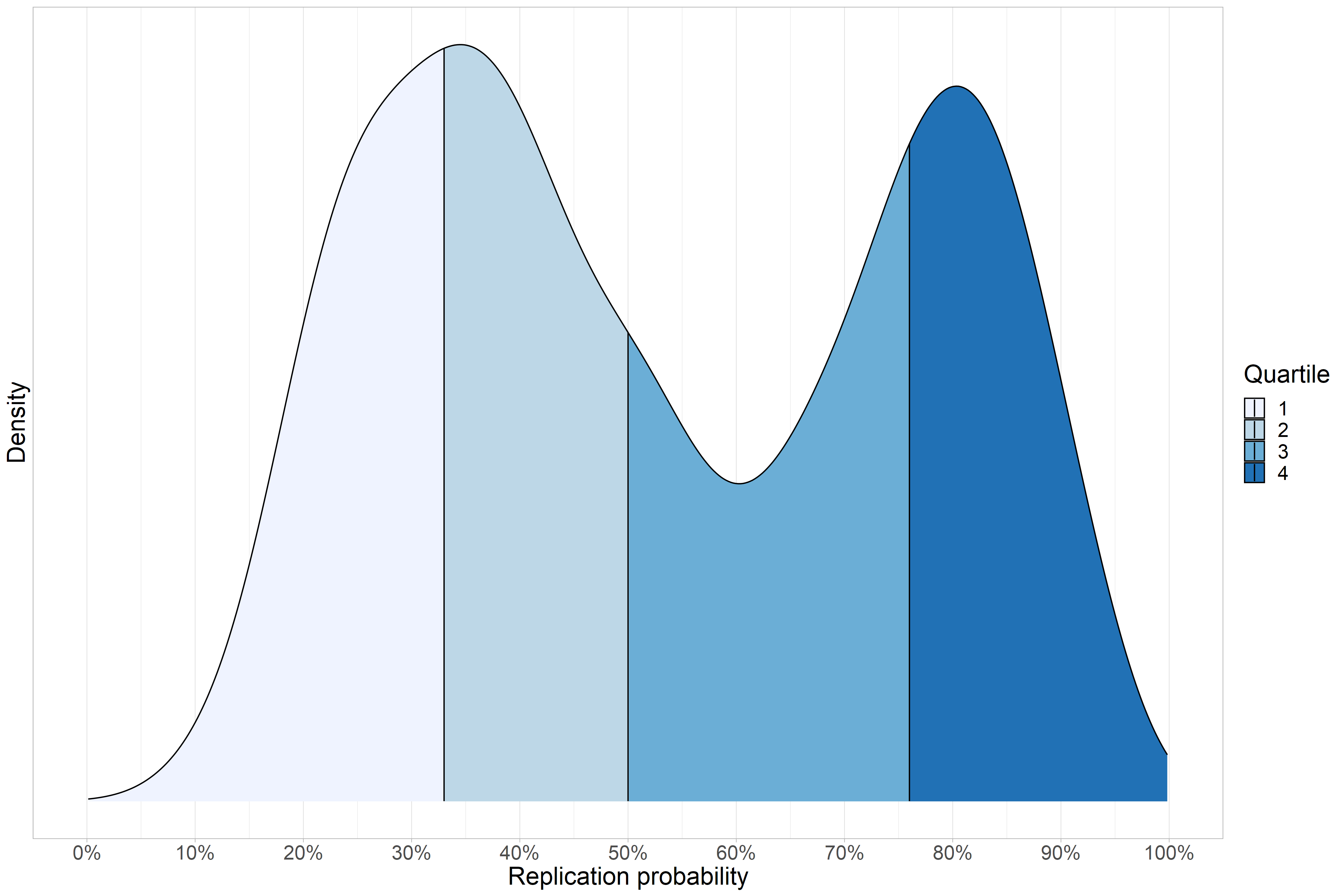
My average forecast was in line with the market. A quarter of the claims were above 76%. And a quarter of them were below 33%: we're talking hundreds upon hundreds of terrible papers, and this is just a tiny sample of the annual academic production.
Criticizing bad science from an abstract, 10000-foot view is pleasant: you hear about some stuff that doesn't replicate, some methodologies that seem a bit silly. "They should improve their methods", "p-hacking is bad", "we must change the incentives", you declare Zeuslike from your throne in the clouds, and then go on with your day.
But actually diving into the sea of trash that is social science gives you a more tangible perspective, a more visceral revulsion, and perhaps even a sense of Lovecraftian awe at the sheer magnitude of it all: a vast landfill—a great agglomeration of garbage extending as far as the eye can see, effluvious waves crashing and throwing up a foul foam of p=0.049 papers. As you walk up to the diving platform, the deformed attendant hands you a pair of flippers. Noticing your reticence, he gives a subtle nod as if to say: "come on then, jump in".
They Know What They're Doing
Prediction markets work well because predicting replication is easy.3 There's no need for a deep dive into the statistical methodology or a rigorous examination of the data, no need to scrutinize esoteric theories for subtle errors—these papers have obvious, surface-level problems.
There's a popular belief that weak studies are the result of unconscious biases leading researchers down a "garden of forking paths". Given enough "researcher degrees of freedom" even the most punctilious investigator can be misled.
I find this belief impossible to accept. The brain is a credulous piece of meat4 but there are limits to self-delusion. Most of them have to know. It's understandable to be led down the garden of forking paths while producing the research, but when the paper is done and you give it a final read-over you will surely notice that all you have is a n=23, p=0.049 three-way interaction effect (one of dozens you tested, and with no multiple testing adjustments of course). At that point it takes more than a subtle unconscious bias to believe you have found something real. And even if the authors really are misled by the forking paths, what are the editors and reviewers doing? Are we supposed to believe they are all gullible rubes?
People within the academy don't want to rock the boat. They still have to attend the conferences, secure the grants, publish in the journals, show up at the faculty meetings: all these things depend on their peers. When criticising bad research it's easier for everyone to blame the forking paths rather than the person walking them. No need for uncomfortable unpleasantries. The fraudster can admit, without much of a hit to their reputation, that indeed they were misled by that dastardly garden, really through no fault of their own whatsoever, at which point their colleagues on twitter will applaud and say "ah, good on you, you handled this tough situation with such exquisite virtue, this is how progress happens! hip, hip, hurrah!" What a ridiculous charade.
Even when they do accuse someone of wrongdoing they use terms like "Questionable Research Practices" (QRP). How about Questionable Euphemism Practices?
- When they measure a dozen things and only pick their outcome variable at the end, that's not the garden of forking paths but the greenhouse of fraud.
- When they do a correlational analysis but give "policy implications" as if they were doing a causal one, they're not walking around the garden, they're doing the landscaping of forking paths.
- When they take a continuous variable and arbitrarily bin it to do subgroup analysis or when they add an ad hoc quadratic term to their regression, they're...fertilizing the garden of forking paths? (Look, there's only so many horticultural metaphors, ok?)
The bottom line is this: if a random schmuck with zero domain expertise like me can predict what will replicate, then so can scientists who have spent half their lives studying this stuff. But they sure don't act like it.
...or Maybe They Don't?
The horror! The horror!
Check out this crazy chart from Yang et al. (2020):

Yes, you're reading that right: studies that replicate are cited at the same rate as studies that do not. Publishing your own weak papers is one thing, but citing other people's weak papers? This seemed implausible, so I decided to do my own analysis with a sample of 250 articles from the Replication Markets project. The correlation between citations per year and (market-estimated) probability of replication was -0.05!
You might hypothesize that the citations of non-replicating papers are negative, but negative citations are extremely rare.5 One study puts the rate at 2.4%. Astonishingly, even after retraction the vast majority of citations are positive, and those positive citations continue for decades after retraction.6
As in all affairs of man, it once again comes down to Hanlon's Razor. Either:
- Malice: they know which results are likely false but cite them anyway.
- or, Stupidity: they can't tell which papers will replicate even though it's quite easy.
Accepting the first option would require a level of cynicism that even I struggle to muster. But the alternative doesn't seem much better: how can they not know? I, an idiot with no relevant credentials or knowledge, can fairly accurately determine good research from bad, but all the tenured experts can not? How can they not tell which papers are retracted?
I think the most plausible explanation is that scientists don't read the papers they cite, which I suppose involves both malice and stupidity.7 Gwern has a nice write-up on this question citing some ingenious analyses based on the proliferation of misprints: "Simkin & Roychowdhury venture a guess that as many as 80% of authors citing a paper have not actually read the original". Once a paper is out there nobody bothers to check it, even though they know there's a 50-50 chance it's false!
Whatever the explanation might be, the fact is that the academic system does not allocate citations to true claims.8 This is bad not only for the direct effect of basing further research on false results, but also because it distorts the incentives scientists face. If nobody cited weak studies, we wouldn't have so many of them. Rewarding impact without regard for the truth inevitably leads to disaster.
There Are No Journals With Strict Quality Standards
Naïvely you might expect that the top-ranking journals would be full of studies that are highly likely to replicate, and the low-ranking journals would be full of p<0.1 studies based on five undergraduates. Not so! Like citations, journal status and quality are not very well correlated: there is no association between statistical power and impact factor, and journals with higher impact factor have more papers with erroneous p-values.
This pattern is repeated in the Replication Markets data. As you can see in the chart below, there's no relationship between h-index (a measure of impact) and average expected replication rates. There's also no relationship between h-index and expected replication within fields.

Even the crème de la crème of economics journals barely manage a ⅔ expected replication rate. 1 in 5 articles in QJE scores below 50%, and this is a journal that accepts just 1 out of every 30 submissions. Perhaps this (partially) explains why scientists are undiscerning: journal reputation acts as a cloak for bad research. It would be fun to test this idea empirically.
Here you can see the distribution of replication estimates for every journal in the RM sample:
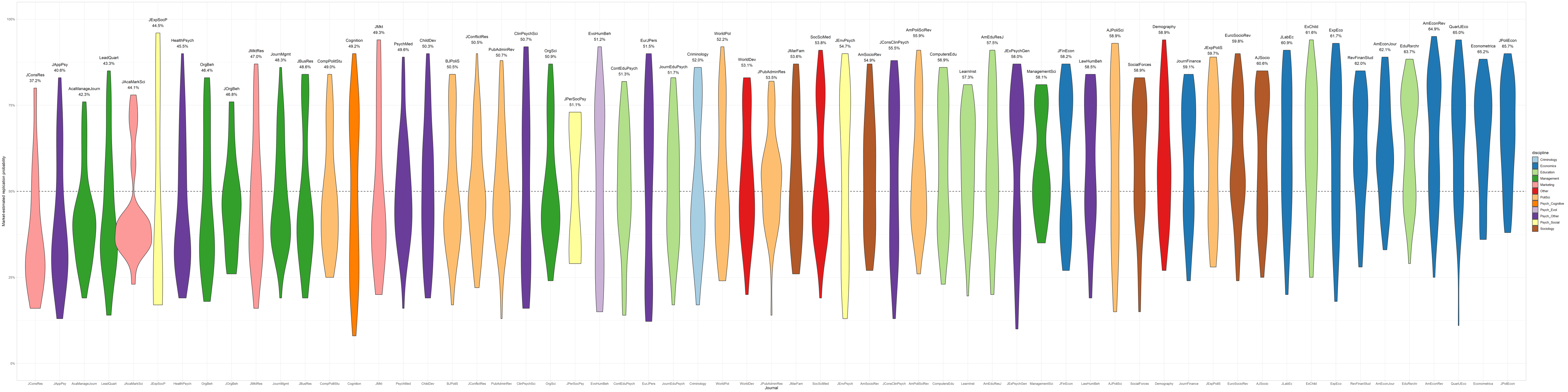
As far as I can tell, for most journals the question of whether the results in a paper are true is a matter of secondary importance. If we model journals as wanting to maximize "impact", then this is hardly surprising: as we saw above, citation counts are unrelated to truth. If scientists were more careful about what they cited, then journals would in turn be more careful about what they publish.
Things Are Not Getting Better
Before we got to see any of the actual Replication Markets studies, we voted on the expected replication rates by year. Gordon et al. (2020) has that data: replication rates were expected to steadily increase from 43% in 2009/2010 to 55% in 2017/2018.
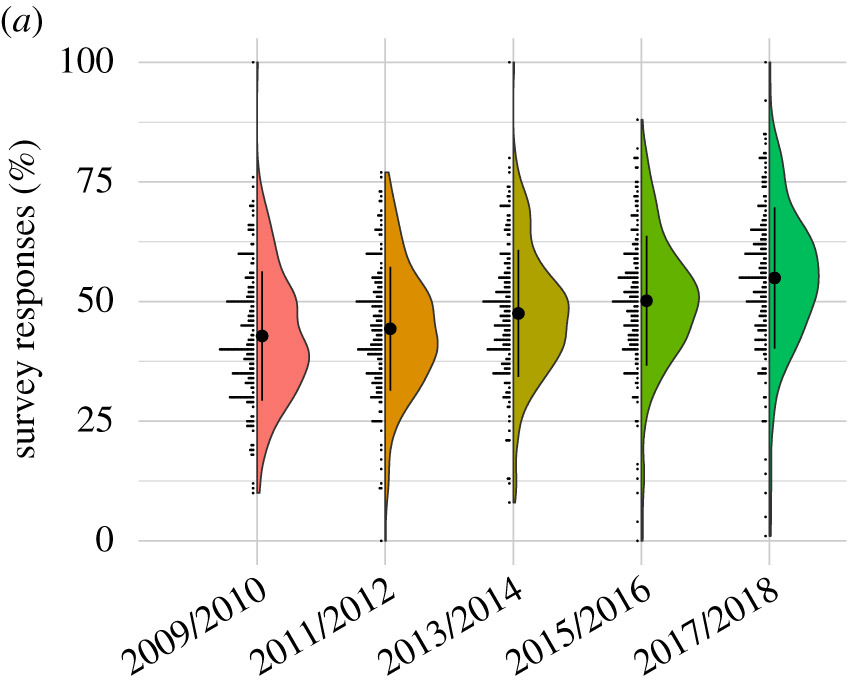
This is what the average predictions looked like after seeing the papers: from 53.4% in 2009 to 55.8% in 2018 (difference not statistically significant; black dots are means).
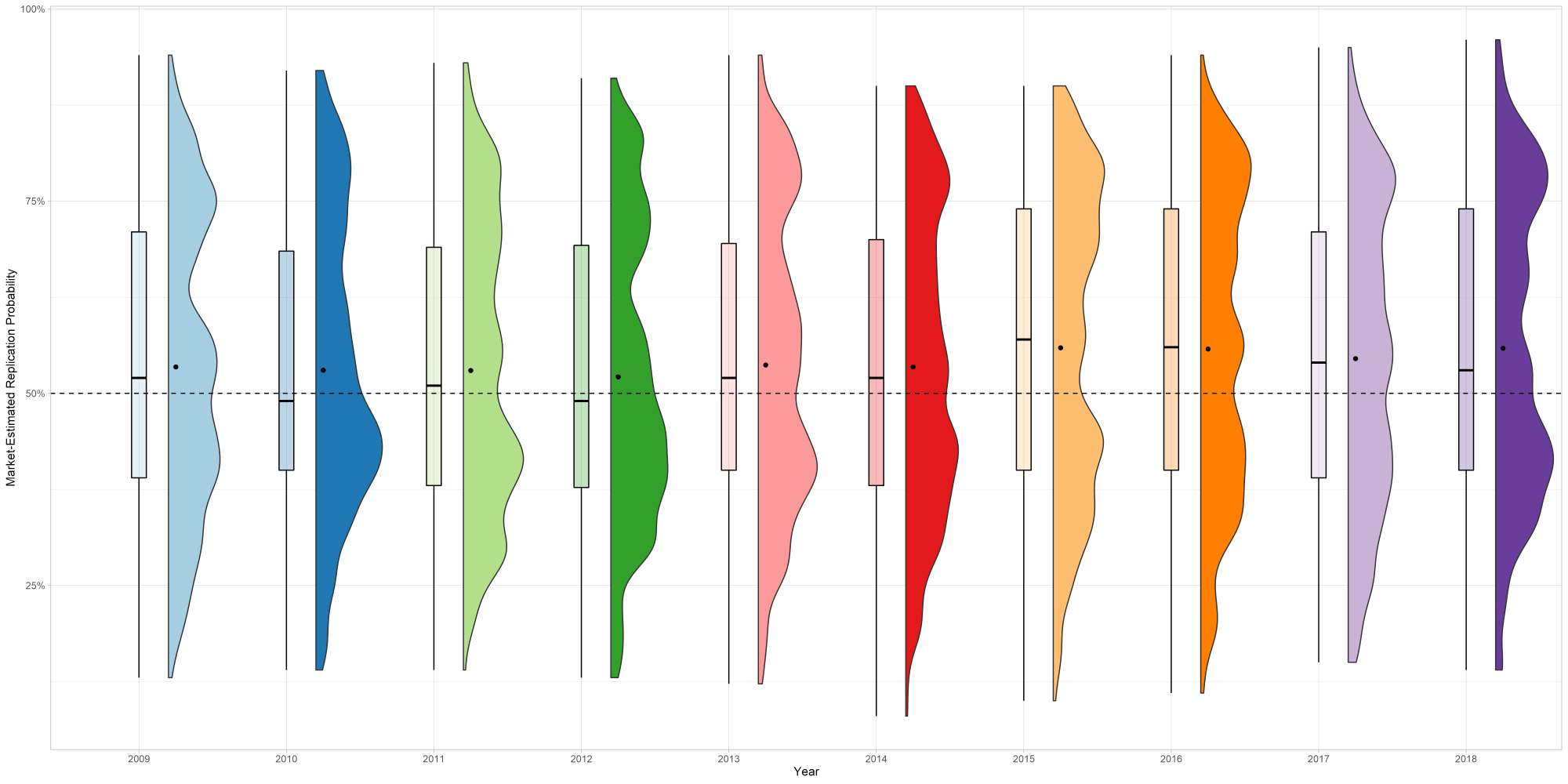
I frequently encounter the notion that after the replication crisis hit there was some sort of great improvement in the social sciences, that people wouldn't even dream of publishing studies based on 23 undergraduates any more (I actually saw plenty of those), etc. Stuart Ritchie's new book praises psychologists for developing "systematic ways to address" the flaws in their discipline. In reality there has been no discernible improvement.
The results aren't out yet, so it's possible that the studies have improved in subtle ways which the forecasters have not been able to detect. Perhaps the actual replication rates will be higher. But I doubt it. Looking at the distribution of p-values over time, there's a small increase in the proportion of p<.001 results, but nothing like the huge improvement that was expected.
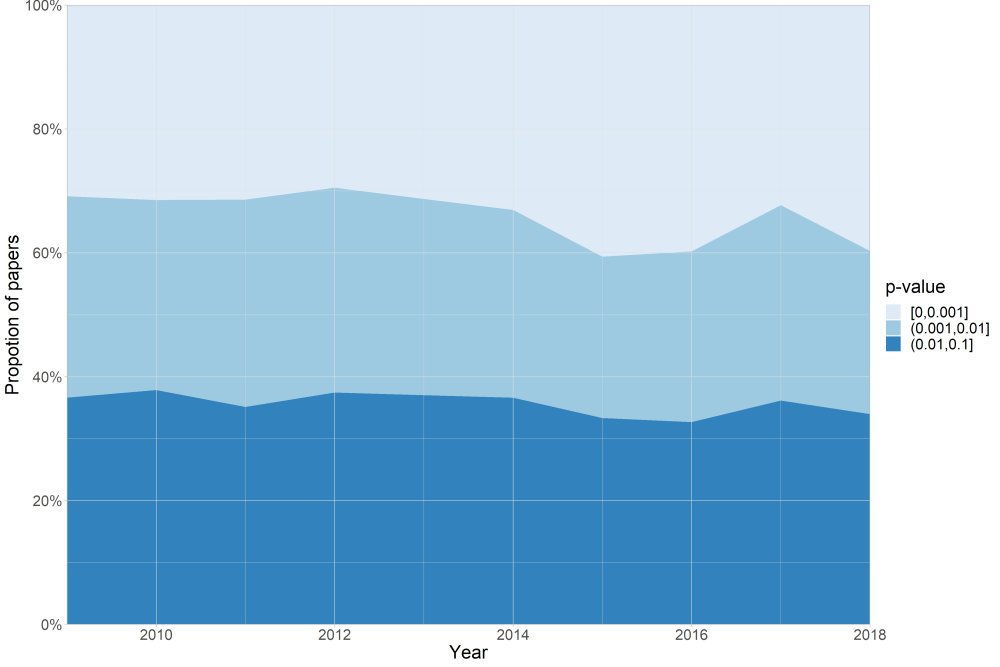
Everyone is Complicit
Authors are just one small cog in the vast machine of scientific production. For this stuff to be financed, generated, published, and eventually rewarded requires the complicity of funding agencies, journal editors, peer reviewers, and hiring/tenure committees. Given the current structure of the machine, ultimately the funding agencies are to blame.9 But "I was just following the incentives" only goes so far. Editors and reviewers don't actually need to accept these blatantly bad papers.
Journals and universities certainly can't blame the incentives when they stand behind fraudsters to the bitter end. Paolo Macchiarini "left a trail of dead patients" but was protected for years by his university. Andrew Wakefield's famously fraudulent autism-MMR study took 12 years to retract. Even when the author of a paper admits the results were entirely based on an error, journals still won't retract.
Elisabeth Bik documents her attempts to report fraud to journals. It looks like this:
The Editor in Chief of Neuroscience Letters [Yale's Stephen G. Waxman] never replied to my email. The APJTM journal had a new publisher, so I wrote to both current Editors in Chief, but they never replied to my email.
Two papers from this set had been published in Wiley journals, Gerodontology and J Periodontology. The EiC of the Journal of Periodontology never replied to my email. None of the four Associate Editors of that journal replied to my email either. The EiC of Gerodontology never replied to my email.
Even when they do take action, journals will often let scientists "correct" faked figures instead of retracting the paper! The rate of retraction is about 0.04%; it ought to be much higher.
And even after being caught for outright fraud, about half of the offenders are allowed to keep working: they "have received over $123 million in federal funding for their post-misconduct research efforts".
Just Because a Paper Replicates Doesn't Mean it's Good
First: a replication of a badly designed study is still badly designed. Suppose you are a social scientist, and you notice that wet pavements tend to be related to umbrella usage. You do a little study and find the correlation is bulletproof. You publish the paper and try to sneak in some causal language when the editors/reviewers aren't paying attention. Rain is never even mentioned. Of course if someone repeats your study, they will get a significant result every time. This may sound absurd, but it describes a large proportion of the papers that successfully replicate.
Economists and education researchers tend to be relatively good with this stuff, but as far as I can tell most social scientists go through 4 years of undergrad and 4-6 years of PhD studies without ever encountering ideas like "identification strategy", "model misspecification", "omitted variable", "reverse causality", or "third-cause". Or maybe they know and deliberately publish crap. Fields like nutrition and epidemiology are in an even worse state, but let's not get into that right now.
"But Alvaro, correlational studies can be usef-" Spare me.
Second: the choice of claim for replication. For some papers it's clear (eg math educational intervention → math scores), but other papers make dozens of different claims which are all equally important. Sometimes the Replication Markets organisers picked an uncontroversial claim from a paper whose central experiment was actually highly questionable. In this way a study can get the "successfully replicates" label without its most contentious claim being tested.
Third: effect size. Should we interpret claims in social science as being about the magnitude of an effect, or only about its direction? If the original study says an intervention raises math scores by .5 standard deviations and the replication finds that the effect is .2 standard deviations (though still significant), that is considered a success that vindicates the original study! This is one area in which we absolutely have to abandon the binary replicates/doesn't replicate approach and start thinking more like Bayesians.
Fourth: external validity. A replicated lab experiment is still a lab experiment. While some replications try to address aspects of external validity (such as generalizability across different cultures), the question of whether these effects are relevant in the real world is generally not addressed.
Fifth: triviality. A lot of the papers in the 85%+ chance-to-replicate range are just really obvious. "Homeless students have lower test scores", "parent wealth predicts their children's wealth", that sort of thing. These are not worthless, but they're also not really expanding the frontiers of science.
So: while about half the papers will replicate, I would estimate that only half of those are actually worthwhile.
Lack of Theory
The majority of journal articles are almost completely atheoretical. Even if all the statistical, p-hacking, publication bias, etc. issues were fixed, we'd still be left with a ton of ad-hoc hypotheses based, at best, on (WEIRD) folk intuitions. But how can science advance if there's no theoretical grounding, nothing that can be refuted or refined? A pile of "facts" does not a progressive scientific field make.
Michael Muthukrishna and the superhuman Joe Henrich have written a paper called A Problem in Theory which covers the issue better than I ever could. I highly recommend checking it out.
Rather than building up principles that flow from overarching theoretical frameworks, psychology textbooks are largely a potpourri of disconnected empirical findings.
There's Probably a Ton of Uncaught Frauds
This is a fairly lengthy topic, so I made a separate post for it. tl;dr: I believe about 1% of falsified/fabricated papers are retracted, but overall they represent a very small portion of non-replicating research.
Power: Not That Bad
[Warning: technical section. Skip ahead if bored.]
A quick refresher on hypothesis testing:
- α, the significance level, is the probability of a false positive.
- β, or type II error, is the probability of a false negative.
- Power is (1-β): if a study has 90% power, there's a 90% chance of successfully detecting the effect being studied. Power increases with sample size and effect size.
- The probability that a significant p-value indicates a true effect is not 1-α. It is called the positive predictive value (PPV), and is calculated as follows:
This great diagram by Felix Schönbrodt gives the intuition behind PPV:
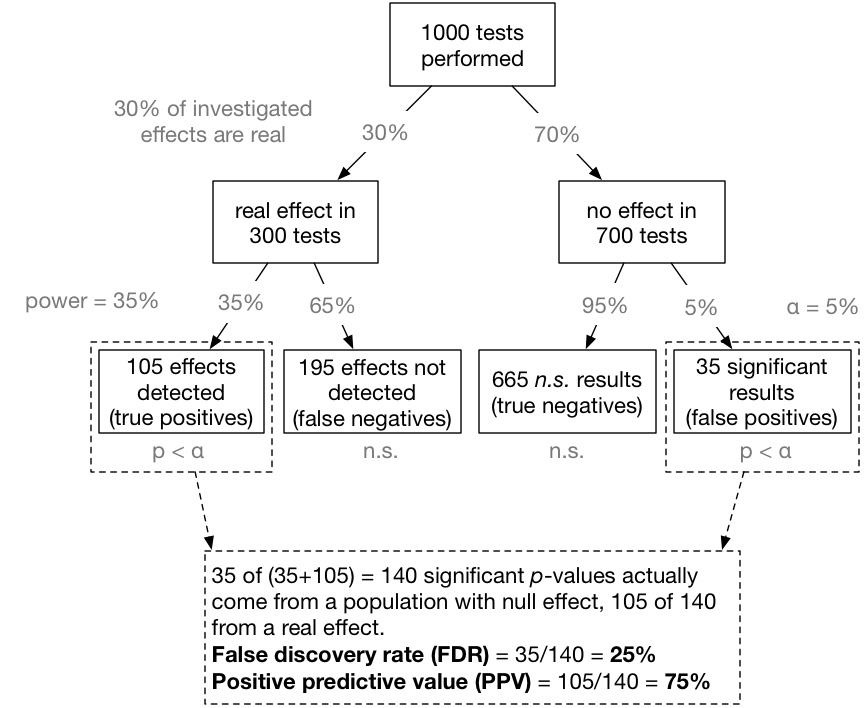
This model makes the assumption that effects can be neatly split into two categories: those that are "real" and those that are not. But is this accurate? In the opposite extreme you have the "crud factor": everything is correlated so if your sample is big enough you will always find a real effect.10 As Bakan puts it: "there is really no good reason to expect the null hypothesis to be true in any population". If you look at the universe of educational interventions, for example, are they going to be neatly split into two groups of "real" and "fake" or is it going to be one continuous distribution? What does "false positive" even mean if there are no "fake" effects, unless it refers purely to the direction of the effect? Perhaps the crud factor is wrong, at least when it comes to causal effects? Perhaps the pragmatic solution is to declare that all effects with, say, d<.1 are fake and the rest are real? Or maybe we should just go full Bayesian?
Anyway, let's pretend the previous paragraph never happened. Where do we find the prior? There are a few different approaches, and they're all problematic.11
- Scheel, Schijen & Lakens (2020) find that 44% of RRs have positive results.12 With an average power of 91.5%, assuming α=5%, working backwards we find that 45% of investigated effects are real.
- Using a z-curves approach to estimate the number of unpublished studies, Ulrich Schimmack estimates a 23% discovery rate in social psychology but this depends on distributional assumptions.
- Ingre & Nilsonne (2018) based on OSC replications (36% replication rate, on the low side) argue for a 5-20% prior, but there are some questionable assumptions there.13
- Meehl says 10% based purely on his intuitions. And if anecdotes like this one are to be believed, it might be even lower in some areas.
The exact number doesn't really matter that much (there's nothing we can do about it), so I'm going to go ahead and use a prior of 25% for the calculations below. The main takeaways don't change with a different prior value.
Now the only thing we're missing is the power of the typical social science study. To determine that we need to know 1) sample sizes (easy), and 2) the effect size of true effects (not so easy).14 I'm going to use the results of extremely high-powered, large-scale replication efforts:
- Many Labs 2 had an average Cohen's d of .62 for the studies with significant effects.
- Camerer et al. (2018) found an average d of .85 for the studies that replicated.
- Open Science Collaboration (2015) had an average d of .93 for the 36% of studies that replicated.
Surprisingly large, right? We can then use the power estimates in Szucs & Ioannidis (2017): they give an average power of .49 for "medium effects" (d=.5) and .71 for "large effects" (d=.8). Let's be conservative and split the difference.
With a prior of 25%, power of 60%, and α=5%, PPV is equal to 80%. Assuming no fraud and no QRPs, 20% of positive findings will be false.
These averages hide a lot of heterogeneity: it's well-established that studies of large effects are adequately powered whereas studies of small effects are underpowered, so the PPV is going to be smaller for small effects. There are also large differences depending on the field you're looking at. The lower the power the bigger the gains to be had from increasing sample sizes.
This is what PPV looks like for the full range of prior/power values, with α=5%:
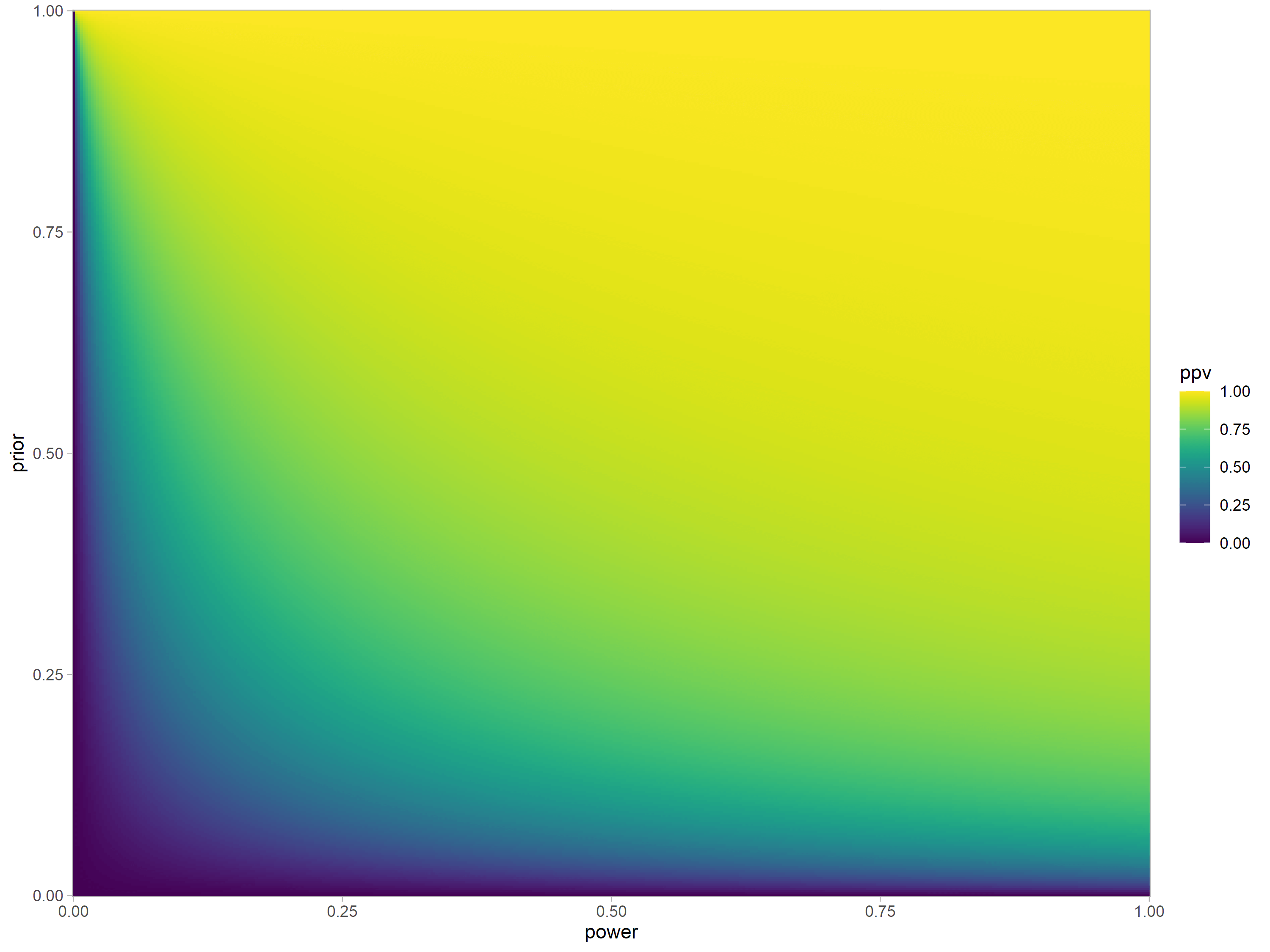
At the current prior/power levels, PPV is more sensitive to the prior: we can only squeeze small gains out of increasing power. That's a bit of a problem given the fact that increasing power is relatively easy, whereas increasing the chance that the effect you're investigating actually exists is tricky, if not impossible. Ultimately scientists want to discover surprising results—in other words, results with a low prior.
I made a little widget so you can play around with the values:
Alpha | 0.05 | |
Power | 0.5 | |
Prior | 0.25 |
False positives | |
True positives | |
False negatives | |
True negatives | |
PPV |
Assuming a 25% prior, increasing power from 60% to 90% would require more than twice the sample size and would only increase PPV by 5.7 percentage points. It's something, but it's no panacea. However, there is something else we could do: sample size is a budget, and we can allocate that budget either to higher power or to a lower significance cutoff. Lowering alpha is far more effective at reducing the false discovery rate.15
Let's take a look at 4 different different power/alpha scenarios, assuming a 25% prior and d=0.5 effect size.16 The required sample sizes are for a one-sided t-test.
False Discovery Rate | |||
---|---|---|---|
α | |||
0.05 | 0.005 | ||
Power | 0.5 | 23.1% | 2.9% |
0.8 | 15.8% | 1.8% |
Required Sample Size | |||
---|---|---|---|
α | |||
0.05 | 0.005 | ||
Power | 0.5 | 45 | 110 |
0.8 | 100 | 190 |
To sum things up: power levels are decent on average and improving them wouldn't do much. Power increases should be focused on studies of small effects. Lowering the significance cutoff achieves much more for the same increase in sample size.
Field of Dreams
Before we got to see any of the actual Replication Markets studies, we voted on the expected replication rates by field. Gordon et al. (2020) has that data:
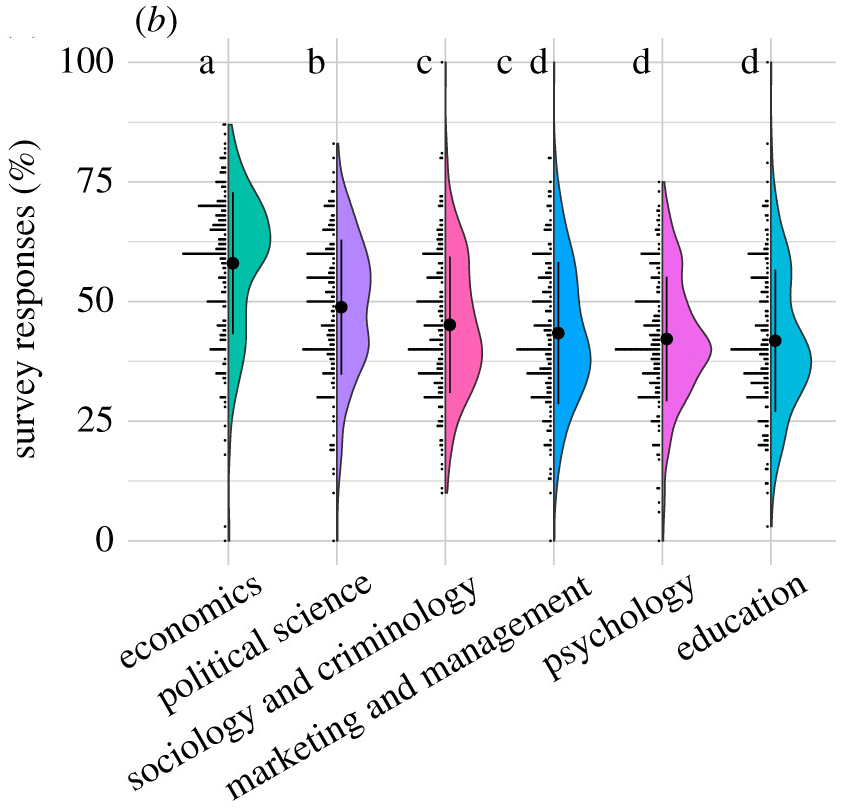
This is what the predictions looked like after seeing the papers:
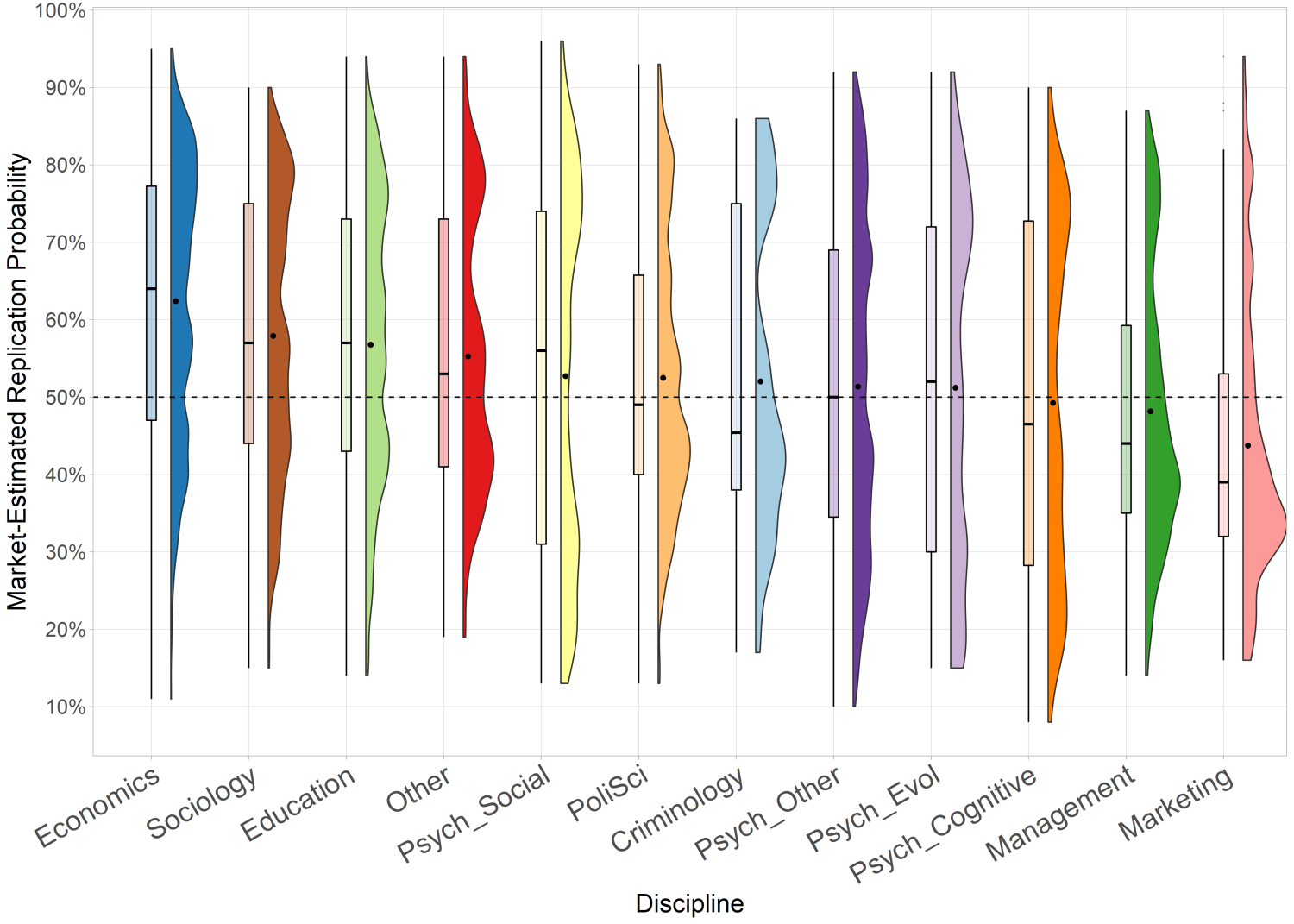
Economics is Predictably Good
Economics topped the charts in terms of expectations, and it was by far the strongest field. There are certainly large improvements to be made—a 2/3 replication rate is not something to be proud of. But reading their papers you get the sense that at least they're trying, which is more than can be said of some other fields. 6 of the top 10 economics journals participated, and they did quite well: QJE is the behemoth of the field and it managed to finish very close to the top. A unique weakness of economics is the frequent use of absurd instrumental variables. I doubt there's anyone (including the authors) who is convinced by that stuff, so let's cut it out.
EvoPsych is Surprisingly Bad
You were supposed to destroy the Sith, not join them!
Going into this, my view of evolutionary psychology was shaped by people like Cosmides, Tooby, DeVore, Boehm, and so on. You know, evolutionary psychology! But the studies I skimmed from evopsych journals were mostly just weak social psychology papers with an infinitesimally thin layer of evolutionary paint on top. Few people seem to take the "evolutionary" aspect really seriously.
Also underdetermination problems are particularly difficult in this field and nobody seems to care.
Education is Surprisingly Good
Education was expected to be the worst field, but it ended up being almost as strong as economics. When it came to interventions there were lots of RCTs with fairly large samples, which made their claims believable. I also got the sense that p-hacking is more difficult in education: there's usually only one math score which measures the impact of a math intervention, there's no early stopping, etc.
However, many of the top-scoring papers were trivial (eg "there are race differences in science scores"), and the field has a unique problem which is not addressed by replication: educational intervention effects are notorious for fading out after a few years. If the replications waited 5 years to follow up on the students, things would look much, much worse.
Demography is Good
Who even knew these people existed? Yet it seems they do (relatively) competent work. googles some of the authors Ah, they're economists. Well.
Criminology Should Just Be Scrapped
If you thought social psychology was bad, you ain't seen nothin' yet. Other fields have a mix of good and bad papers, but criminology is a shocking outlier. Almost every single paper I read was awful. Even among the papers that are highly likely to replicate, it's de rigueur to confuse correlation for causation.
If we compare criminology to, say, education, the headline replication rates look similar-ish. But the designs used in education (typically RCT, diff-in-diff, or regression discontinuity) are at least in principle capable of detecting the effects they're looking for. That's not really the case for criminology. Perhaps this is an effect of the (small number of) specific journals selected for RM, and there is more rigorous work published elsewhere.
There's no doubt in my mind that the net effect of criminology as a discipline is negative: to the extent that public policy is guided by these people, it is worse. Just shameful.
Marketing/Management
In their current state these are a bit of a joke, but I don't think there's anything fundamentally wrong with them. Sure, some of the variables they use are a bit fluffy, and of course there's a lack of theory. But the things they study are a good fit for RCTs, and if they just quintupled their sample sizes they would see massive improvements.
Cognitive Psychology
Much worse than expected; generally has a reputation as being one of the more solid subdisciplines of psychology, and has done well in previous replication projects. Not sure what went wrong here. It's only 50 papers and they're all from the same journal, so perhaps it's simply an unrepresentative sample.
Social Psychology
More or less as expected. All the silly stuff you've heard about is still going on.
Limited Political Hackery
Some of the most highly publicized social science controversies of the last decade happened at the intersection between political activism and low scientific standards: the implicit association test,17 stereotype threat, racial resentment, etc. I thought these were representative of a wider phenomenon, but in reality they are exceptions. The vast majority of work is done in good faith.
While blatant activism is rare, there is a more subtle background ideological influence which affects the assumptions scientists make, the types of questions they ask, and how they go about testing them. It's difficult to say how things would be different under the counterfactual of a more politically balanced professoriate, though.
Interaction Effects Bad
A paper whose main finding is an interaction effect is about 10 percentage points less likely to replicate. Their usage is not inherently wrong, sometimes it's theoretically justified. But all too often you'll see blatant fishing expeditions with a dozen double and triple ad hoc interactions thrown into the regression. They make it easy to do naughty things and tend to be underpowered.
Nothing New Under the Sun
All is mere breath, and herding the wind.
The replication crisis did not begin in 2010, it began in the 1950s. All the things I've written above have been written before, by respected and influential scientists. They made no difference whatsoever. Let's take a stroll through the museum of metascience.
Sterling (1959) analyzed psychology articles published in 1955-56 and noted that 97% of them rejected their null hypothesis. He found evidence of a huge publication bias, and a serious problem with false positives which was compounded by the fact that results are "seldom verified by independent replication".
Nunnally (1960) noted various problems with null hypothesis testing, underpowered studies, over-reliance on student samples (it doesn't take Joe Henrich to notice that using Western undergrads for every experiment might be a bad idea), and much more. The problem (or excuse) of publish-or-perish, which some portray as a recent development, was already in place by this time.18
The "reprint race" in our universities induces us to publish hastily-done, small studies and to be content with inexact estimates of relationships.
Jacob Cohen (of Cohen's d fame) in a 1962 study analyzed the statistical power of 70 psychology papers: he found that underpowered studies were a huge problem, especially for those investigating small effects. Successive studies by Sedlemeier & Gigerenzer in 1989 and Szucs & Ioannidis in 2017 found no improvement in power.
If we then accept the diagnosis of general weakness of the studies, what treatment can be prescribed? Formally, at least, the answer is simple: increase sample sizes.
Paul Meehl (1967) is highly insightful on problems with null hypothesis testing in the social sciences, the "crud factor", lack of theory, etc. Meehl (1970) brilliantly skewers the erroneous (and still common) tactic of automatically controling for "confounders" in observational designs without understanding the causal relations between the variables. Meehl (1990) is downright brutal: he highlights a series issues which, he argues, make psychological theories "uninterpretable". He covers low standards, pressure to publish, low power, low prior probabilities, and so on.
I am prepared to argue that a tremendous amount of taxpayer money goes down the drain in research that pseudotests theories in soft psychology and that it would be a material social advance as well as a reduction in what Lakatos has called “intellectual pollution” if we would quit engaging in this feckless enterprise.
Rosenthal (1979) covers publication bias and the problems it poses for meta-analyses: "only a few studies filed away could change the combined significant result to a nonsignificant one". Cole, Cole & Simon (1981) present experimental evidence on the evaluation of NSF grant proposals: they find that luck plays a huge factor as there is little agreement between reviewers.
I could keep going to the present day with the work of Goodman, Gelman, Nosek, and many others. There are many within the academy who are actively working on these issues: the CASBS Group on Best Practices in Science, the Meta-Research Innovation Center at Stanford, the Peer Review Congress, the Center for Open Science. If you click those links you will find a ton of papers on metascientific issues. But there seems to be a gap between awareness of the problem and implementing policy to fix it. You've got tons of people doing all this research and trying to repair the broken scientific process, while at the same time journal editors won't even retract blatantly fraudulent research.
There is even a history of government involvement. In the 70s there were battles in Congress over questionable NSF grants, and in the 80s Congress (led by Al Gore) was concerned about scientific integrity, which eventually led to the establishment of the Office of Scientific Integrity. (It then took the federal government another 11 years to come up with a decent definition of scientific misconduct.) After a couple of embarrassing high-profile prosecutorial failures they more or less gave up, but they still exist today and prosecute about a dozen people per year.
Generations of psychologists have come and gone and nothing has been done. The only difference is that today we have a better sense of the scale of the problem. The one ray of hope is that at least we have started doing a few replications, but I don't see that fundamentally changing things: replications reveal false positives, but they do nothing to prevent those false positives from being published in the first place.
What To Do
The reason nothing has been done since the 50s, despite everyone knowing about the problems, is simple: bad incentives. The best cases for government intervention are collective action problems: situations where the incentives for each actor cause suboptimal outcomes for the group as a whole, and it's difficult to coordinate bottom-up solutions. In this case the negative effects are not confined to academia, but overflow to society as a whole when these false results are used to inform business and policy.
Nobody actually benefits from the present state of affairs, but you can't ask isolated individuals to sacrifice their careers for the "greater good": the only viable solutions are top-down, which means either the granting agencies or Congress (or, as Scott Alexander has suggested, a Science Czar). You need a power that sits above the system and has its own incentives in order: this approach has already had success with requirements for pre-registration and publication of clinical trials. Right now I believe the most valuable activity in metascience is not replication or open science initiatives but political lobbying.19
- Earmark 60% of funding for registered reports (ie accepted for publication based on the preregistered design only, not results). For some types of work this isn't feasible, but for ¾ of the papers I skimmed it's possible. In one fell swoop, p-hacking and publication bias would be virtually eliminated.20
- An NSF/NIH inquisition that makes sure the published studies match the pre-registration (there's so much """"""""""QRP"""""""""" in this area you wouldn't believe). The SEC has the power to ban people from the financial industry—let's extend that model to academia.21
- Earmark 10% of funding for replications. When the majority of publications are registered reports, replications will be far less valuable than they are today. However, intelligently targeted replications still need to happen.
- Earmark 1% of funding for progress studies. Including metascientific research that can be used to develop a serious science policy in the future.
- Increase sample sizes and lower the significance threshold to .005. This one needs to be targeted: studies of small effects probably need to quadruple their sample sizes in order to get their power to reasonable levels. The median study would only need 2x or so. Lowering alpha is generally preferable to increasing power. "But Alvaro, doesn't that mean that fewer grants would be funded?" Yes.
- Ignore citation counts. Given that citations are unrelated to (easily-predictable) replicability, let alone any subtler quality aspects, their use as an evaluative tool should stop immediately.
- Open data, enforced by the NSF/NIH. There are problems with privacy but I would be tempted to go as far as possible with this. Open data helps detect fraud. And let's have everyone share their code, too—anything that makes replication/reproduction easier is a step in the right direction.
- Financial incentives for universities and journals to police fraud. It's not easy to structure this well because on the one hand you want to incentivize them to minimize the frauds published, but on the other hand you want to maximize the frauds being caught. Beware Goodhart's law!
- Why not do away with the journal system altogether? The NSF could run its own centralized, open website; grants would require publication there. Journals are objectively not doing their job as gatekeepers of quality or truth, so what even is a journal? A combination of taxonomy and reputation. The former is better solved by a simple tag system, and the latter is actually misleading. Peer review is unpaid work anyway, it could continue as is. Attach a replication prediction market (with the estimated probability displayed in gargantuan neon-red font right next to the paper title) and you're golden. Without the crutch of "high ranked journals" maybe we could move to better ways of evaluating scientific output. No more editors refusing to publish replications. You can't shift the incentives: academics want to publish in "high-impact" journals, and journals want to selectively publish "high-impact" research. So just make it impossible. Plus as a bonus side-effect this would finally sink Elsevier.
- Have authors bet on replication of their research. Give them fixed odds, say 1:4—if it's good work, it's +EV for them. This sounds a bit distasteful, so we could structure the same cashflows as a "bonus grant" from the NSF when a paper you wrote replicates successfully.22
And a couple of points that individuals can implement today:
- Just stop citing bad research, I shouldn't need to tell you this, jesus christ what the fuck is wrong with you people.
- Read the papers you cite. Or at least make your grad students to do it for you. It doesn't need to be exhaustive: the abstract, a quick look at the descriptive stats, a good look at the table with the main regression results, and then a skim of the conclusions. Maybe a glance at the methodology if they're doing something unusual. It won't take more than a couple of minutes. And you owe it not only to SCIENCE!, but also to yourself: the ability to discriminate between what is real and what is not is rather useful if you want to produce good research.23
- When doing peer review, reject claims that are likely to be false. The base replication rate for studies with p>.001 is below 50%. When reviewing a paper whose central claim has a p-value above that, you should recommend against publication unless the paper is exceptional (good methodology, high prior likelihood, etc.)24 If we're going to have publication bias, at least let that be a bias for true positives. Remember to subtract another 10 percentage points for interaction effects. You don't need to be complicit in the publication of false claims.
- Stop assuming good faith. I'm not saying every academic interaction should be hostile and adversarial, but the good guys are behaving like dodos right now and the predators are running wild.
...My Only Friend, The End
The first draft of this post had a section titled "Some of My Favorites", where I listed the silliest studies in the sample. But I removed it because I don't want to give the impression that the problem lies with a few comically bad papers in the far left tail of the distribution. The real problem is the median.
It is difficult to convey just how low the standards are. The marginal researcher is a hack and the marginal paper should not exist. There's a general lack of seriousness hanging over everything—if an undergrad cites a retracted paper in an essay, whatever; but if this is your life's work, surely you ought to treat the matter with some care and respect.
Why is the Replication Markets project funded by the Department of Defense? If you look at the NSF's 2019 Performance Highlights, you'll find items such as "Foster a culture of inclusion through change management efforts" (Status: "Achieved") and "Inform applicants whether their proposals have been declined or recommended for funding in a timely manner" (Status: "Not Achieved"). Pusillanimous reports repeat tired clichés about "training", "transparency", and a "culture of openness" while downplaying the scale of the problem and ignoring the incentives. No serious actions have followed from their recommendations.
It's not that they're trying and failing—they appear to be completely oblivious. We're talking about an organization with an 8 billion dollar budget that is responsible for a huge part of social science funding, and they can't manage to inform people that their grant was declined! These are the people we must depend on to fix everything.
When it comes to giant bureaucracies it can be difficult to know where (if anywhere) the actual power lies. But a good start would be at the top: NSF director Sethuraman Panchanathan, SES division director Daniel L. Goroff, NIH director Francis S. Collins, and the members of the National Science Board. The broken incentives of the academy did not appear out of nowhere, they are the result of grant agency policies. Scientists and the organizations that represent them (like the AEA and APA) should be putting pressure on them to fix this ridiculous situation.
The importance of metascience is inversely proportional to how well normal science is working, and right now it could use some improvement. The federal government spends about $100b per year on research, but we lack a systematic understanding of scientific progress, we lack insight into the forces that underlie the upward trajectory of our civilization. Let's take 1% of that money and invest it wisely so that the other 99% will not be pointlessly wasted. Let's invest it in a robust understanding of science, let's invest it in progress studies, let's invest it in—the future.
Thanks to Alexey Guzey and Dormin for their feedback. And thanks to the people at SCORE and the Replication Markets team for letting me use their data and for running this unparalleled program.
- 1.Dreber et al. (2015), Using prediction markets to estimate the reproducibility of scientific research.
Camerer et al. (2018), Evaluating the replicability of social science experiments in Nature and Science between 2010 and 2015. ↩ - 2.The distribution is bimodal because of the way p-values are typically reported: there's a huge difference between p<.01 and p<.001. If actual p-values were reported instead of cutoffs, the distribution would be unimodal. ↩
- 3.Even laypeople are half-decent at it. ↩
- 4.Ludwik Fleck has an amusing bit on the development of anatomy: "Simple lack of 'direct contact with nature' during experimental dissection cannot explain the frequency of the phrase "which becomes visible during autopsy" often accompanying what to us seem the most absurd assertions." ↩
- 5.Another possible explanation is that importance is inversely related to replication probability. In my experience that is not the case, however. If anything it's the opposite: important effects tend to be large effects, and large effects tend to replicate. In general, any "conditioning on a collider"-type explanation doesn't work here because these citations also continue post-retraction. ↩
- 6.Some more:
- "The huge majority of the [post-retraction] citations are positive".
- No significant difference in citations pre- and post-retraction.
- And some more hopeful results from two epidemiology studies: over time they got fewer citations, and negative citations grew to 33%.
- 7.Though I must admit that after reading the papers myself I understand why they would shy away from the task. ↩
- 8.I can tell you what is rewarded with citations though: papers in which the authors find support for their hypothesis. ↩
- 9.Perhaps I don't understand the situation at places like the NSF or the ESRC but the problem seems to be incompetence (or a broken bureaucracy?) rather than misaligned incentives. ↩
- 10.Theoretically there's the possibility of overpowered studies being a problem. Meehl (1967) argues that 1) everything in psychology is correlated (the "crud factor"), and 2) theories only make directional predictions (as opposed to point predictions in eg physics). So as power increases the probability of finding a significant result for a directional prediction approaches 50% regardless of what you're studying. ↩
- 11.In medicine there are plenty of cohort-based publication bias analyses, but I don't think we can generalize from those to the social sciences. ↩
- 12.But RRs are probably not representative of the literature, so this is an overestimate. And who knows how many unpublished pilot studies are behind every RR? ↩
- 13.Dreber et al. (2015) use prediction market probabilities and work backward to get a prior of 9%, but this number is based on unreasonable assumptions about false positives: they don't take into account fraud and QRPs. If priors were really that low, the entire replication crisis would be explained purely by normal sampling error: no QRPs! ↩
- 14.Part of the issue is that the literature is polluted with a ton of false results, which actually pushes estimates of true effect sizes downwards. There's an unfortunate tendency to lump together effect sizes of real and non-existent effects (eg Many Labs 2: "ds were 0.60 for the original findings and 0.15 for the replications"), but that's a meaningless number. ↩
- 15.False negatives are bad too, but they're not as harmful as false positives. Especially since they're almost never published. Also, there's been a ton of stuff written on lowering alpha, a good starting point is Redefine Statistical Significance. ↩
- 16.These figures actually understate the benefit of a lower alpha, because it would also change the calculus around p-hacking. With an alpha of 5%, getting a false positive is quite easy. Simply stopping data collection once you have a significant result has a hit rate of over 20%! Add some dredging and HARKing to that and you can squeeze a result out of anything. With a lower alpha, the chances of p-hacking success will be vastly lower and some researchers won't even bother trying. ↩
- 17.The original IAT paper is worth revisiting. You only really need to read page 1475. The construct validity evidence is laughable. The whole thing is based on N=26 and they find no significant correlation between the IAT and explicit measures of racism. But that's OK, Greenwald says, because the IAT is meant to find secret racists ("reveal explicitly disavowed prejudice")! The question of why a null correlation between implicit and explicit racial attitudes is to be expected is left as an exercise to the reader. The correlation between two racial IATs (male and female names) is .46 and they conveniently forget to mention the comically low test-retest reliability. That's all you need for 13k citations and a consulting industry selling implicit bias to the government for millions of dollars. ↩
- 18.I suspect psychologists today would laugh at the idea of the 1960s being an over-competitive environment. Personally I highly doubt that this situation can be blamed on high (or increasing) productivity. ↩
- 19.You might ask: well, why haven't the independent grant agencies already fixed the problem then? I'm not sure if it's a lack of competence, or caring, or power, or something else. But I find Garrett Jones' arguments on the efficacy of independent government agencies convincing: this model works well in other areas. ↩
- 20."But Alvaro, what if I make an unexpected discovery during my investigation?" Well, you start writing a new registered report, and perhaps publish it as an exploratory result. You may not like it, but that's how we protect against false positives. In cases where only one dataset is available (eg historical data) we must rely on even stricter standards of evidence, to protect against multiple testing. ↩
- 21.Another idea to steal from the SEC: whistleblower rewards. ↩
- 22.This would be immediately exploited by publishing a bunch of trivial results. But that's a solvable problem. In any case, it's much better to have systematic, automatic mechanisms instead of relying on subjective factors and prosecuting of individual cases. ↩
- 23.I believe the SCORE program intends to use the data from Replication Markets to train a ML model that predicts replicability. If scientists had the ability to just run that on every reference in their papers, perhaps they could go back to not reading what they cite. ↩
- 24.Looking at Replication Markets data, about 1 in 4 studies with p>.001 had more than a 50% chance to replicate. Of course I'd consider 50-50 odds far too low a threshold, but you have to start somewhere. "But Alvaro, science is not done paper by paper, it is a cumulative enterprise. We should publish marginal results, even if they're probably not true. They are pieces of evidence that, brick by brick, raise the vast edifice that we call scientific knowledge". In principle this is a good argument: publish everything and let the meta-analyses sort it out. But given the reality of publication bias we must be selective. If registered reports became the standard, this problem would not exist. ↩